January 25, 2019
Success Factors for Industrial Data Science Projects
Speaker: Prof. Dr.-Ing. Jochen Deuse, Institut of Production Systems, TU Dortmund University
The modern industrial production environment receives strong impulses through an ever increasing use of data science methods for optimization purposes. In particular, the consideration of three essential success factors is of great importance for the efficient implementation of such industrial data science projects. Firstly, the project-internal procedure must be aligned with a structured procedure model, such as the CRISP-DM. Secondly, the project team has to be sufficiently interdisciplinary, for example ensuring sufficient domain knowledge. Thirdly, it is necessary to ensure sufficient data quality and quantity for the purposes of analysis, with the support, for example, of using so-called maturity models.
Prof. Dr.-Ing. Jochen Deuse
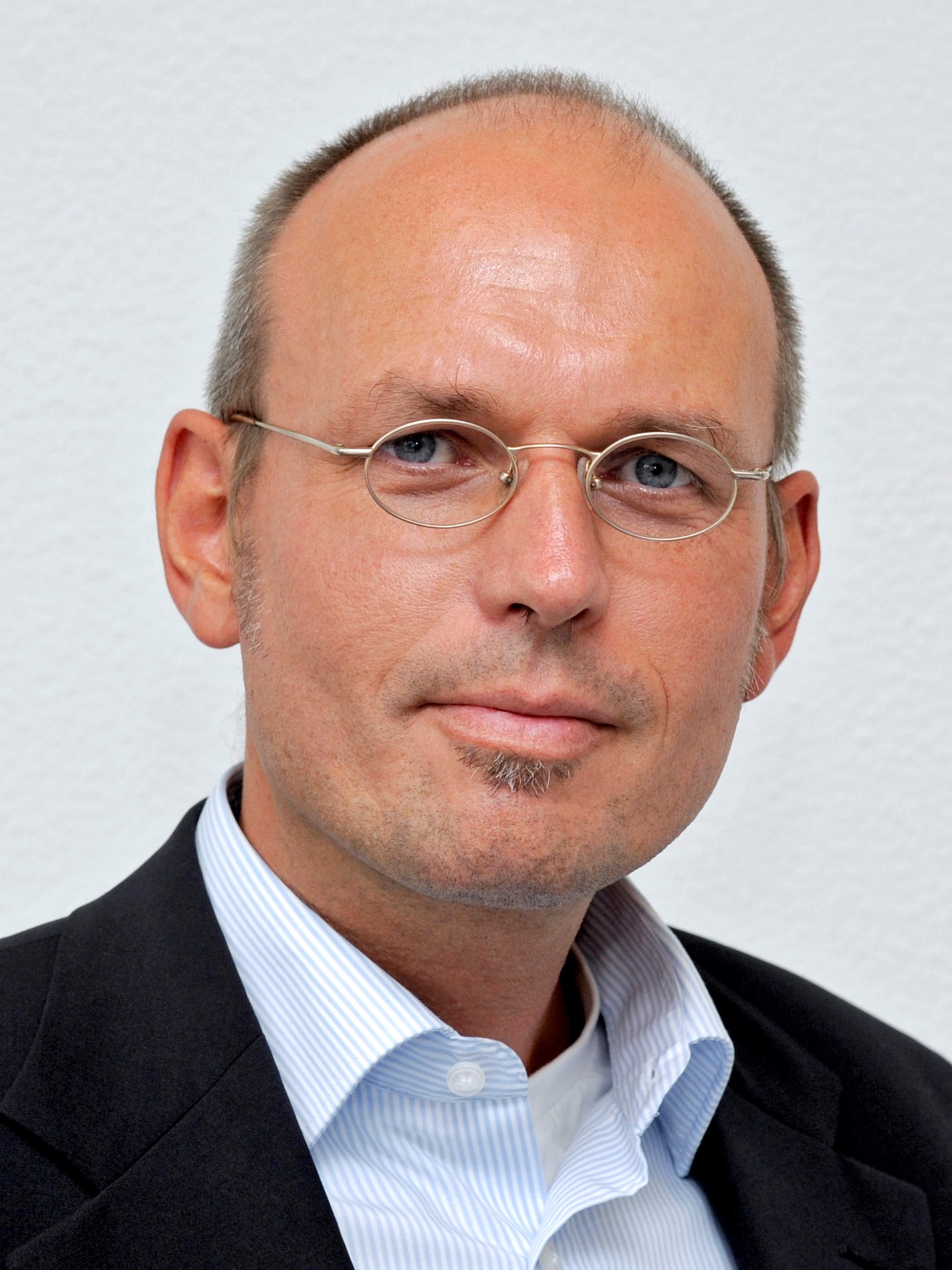
Head of the Institute of Production Systems (IPS), TU Dortmund University
Prof. Dr.-Ing. Jochen Deuse, born in 1967, studied Mechanical Engineering at Dortmund University, Germany, and Manufacturing Technology at the University of Limerick, Ireland. Afterwards he gained his doctorate in engineering (Dr.-Ing.) at the Laboratory for Machine Tools and Production Engineering (WZL) at RWTH Aachen University, Germany. Throughout his career, he held senior management positions in the Bosch Group in Germany and Australia, before joining the Technical University of Dortmund, Germany, to become head of the chair of Industrial Engi- neering in 2005. In 2012 the chair of Industrial Engineering and the chair of Industri- al Robotics and Production Automation merged to form the Institute of Production Systems (IPS) under the direction of Prof. Deuse. Prof. Deuse is also a member of several scientific networks and research associations and a member of the board at the industry network NIRO e.V.